Embracing uncertainty as the target of prediction
Benjamin Van Durme / John Hopkins University
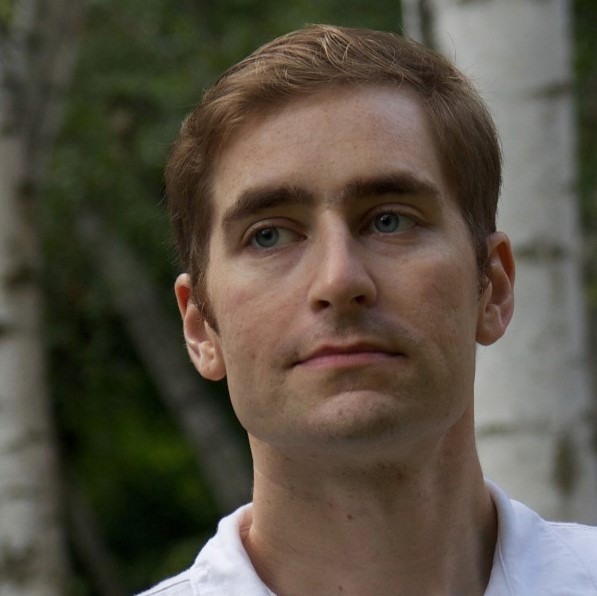
Talk: , -
Abstract:
The Information Extraction and Computational Semantics communities are largely dominated by resources and models that strive for extracting what an observation categorically supports as a true prediction. E.g., "this image is of a CAT", or "that token sequence refers to an ORGANIZATION", or "this sentence ENTAILS that other sentence". As humans we recognize that observations can be ambiguous as to what predictions they evince, but we seem to forget that when building datasets, and then blindly aim to reproduce those annotations when building models. I will discuss a series of projects that explore annotating and modeling subjective likelihood assessments, with a focus on tasks such as semantic parsing and textual entailment. For example, the sentence "Someone is walking a dog in a park" may be interpreted as strong evidence for, "The dog is alive", weak evidence for, "The sun is shining", and cast doubt on (but not strictly "contradict"), "The park is on fire". While our work has concentrated on text, the point applies broadly: how often have you done an image captcha and had hesitation on whether that one picture contained a "bridge"? Let's agree that sometimes the right answer is "maybe".
Bio: Benjamin Van Durme is an Associate Professor of Computer Science at Johns Hopkins University and a Principal Research Scientist at Microsoft Semantic Machines. His research focusses on resources and models for natural language understanding. His observations on the problem of reporting bias in common sense knowledge acquisition was recognized with a best paper award at AKBC 2013.
Bio: Benjamin Van Durme is an Associate Professor of Computer Science at Johns Hopkins University and a Principal Research Scientist at Microsoft Semantic Machines. His research focusses on resources and models for natural language understanding. His observations on the problem of reporting bias in common sense knowledge acquisition was recognized with a best paper award at AKBC 2013.